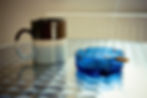
Note: This is the second post in a series on interpreting scientific data. The first post covers statistical significance and p-values.
If you engage with health research on a regular basis, you have probably come across the term ‘confounding.’ It often appears in the ‘methods’ or ‘limitations’ sections of journal articles when statistical models have been used to draw inferences about the data, particularly if those inferences are causal in nature.
Because context is always key, let’s first take a step back and consider exactly what a statistical model is and what it does. Returning to a key concept from the first post in this series, we use statistics to help determine our level of certainty about our observations; in other words, that they are a reflection of a true effect, as opposed to a mere coincidence. Statistical models allow us to do just that and more–we can examine numerous variables and determine how they affect a particular outcome, and how they interact with each other, if necessary. That’s a very simple explanation, and of course you can do much more with statistical models; but basically, a statistical model is a set of equations that best represent the relationship between your variables and your outcome of interest.
Imagine that out of a random sample of 100 people, you took everyone’s height and weight. Those are now our variables. If we were to plot every observation in our dataset (each height and weight measure for all 100 members of our sample), it might look something like this:

Just by eyeballing the chart, it’s pretty clear that there is a relationship between the two variables; as height increases, weight also appears to increase. This is a linear trend, because we could draw a line that would approximate the relationship that we can see in the chart. In other words, our next step is to draw a line that minimizes the distance between itself and each of the 100 observations. This line can be generated from a unique equation that represents the relationship between weight and height as it appears in our sample. We’ve now done simple linear regression, and this is the foundation of most statistical models that are used in the health sciences. From here, we can add even more variables and expand our equation, if we would like.
So what does this have to do with confounding? First, let’s consider the commonplace meaning of the word: to confound is to confuse, muddle, mix-up, or otherwise obscure. This is essentially what we mean when we talk about confounding in a statistical sense as well. If data are ‘confounded,’ it means that some other factor is either concealing, exaggerating, or otherwise distorting a relationship between an exposure and an outcome that we wish to understand. In other words, one way to think of confounding is as a form of interference.
To illustrate, let’s look at a classic example that is often used in epidemiology textbooks: the relationship between coffee consumption and heart disease. Look at the example chart above again, but imagine heart disease replacing weight (our outcome always goes on the Y-axis) and amount of coffee consumed per day replacing height. It would then appear that the more coffee one consumes, the more likely it is that they suffer from heart disease. This suggests that perhaps drinking coffee is causing heart disease. But what if there’s another factor that we didn’t consider?
Let’s draw out the relationship we believe we are seeing between coffee and heart disease:

But what if, in addition to drinking their morning coffee, the people in our sample were also more likely to smoke cigarettes? In other words, what if smoking is associated with coffee drinking? This would be a problem, because we know that smoking is a major risk factor for heart disease. So if more smokers drink coffee than non-smokers, it will appear to us when we plot the data that coffee drinking and heart disease are associated, even though in reality, what we are actually seeing is the relationship between smoking and heart disease.
Let’s draw a new diagram to show what’s going on here:

Now we can see the problem. In this case, smoking is a confounder of the relationship between coffee and heart disease, because it is associated with both the exposure and the outcome. Fortunately, there are ways we can account for this interference in our statistical model–but only if we know who smokes and who doesn’t in our sample. When we do this, we say we are ‘controlling for confounding.’ That’s why it’s so important to measure variables that we think might confound the relationship between our exposure (X) and our outcome (Y)--because in the real world, there are confounders everywhere!
When a study is accused of not controlling for confounding, it means that the authors did not factor confounding variables into their model, and therefore the results cannot be interpreted because we do not know what effect those other variables may be having on our relationship of interest. You might now ask, but how do we know what variables to include as confounders? And the answer is, sometimes we don’t! But usually we can make some educated guesses based on context. That’s why we typically collect a lot of information about our study participants, if we can–so that we can test which variables are confounders, and then account for them accordingly. But sometimes we don’t know very much about our participants, and in that situation, the study authors should acknowledge the possibility of confounding in their limitations section.
You might wonder at this point if all studies involving human beings are confounded–and you’d be right to. Most studies are confounded to an extent, and we often acknowledge the possibility of unmeasured confounding as well–the confounding variables that we have not even thought of yet. But that doesn’t mean that observational studies are worthless, just that we need to consider them in context. To use a familiar adage, it’s best to take everything with a grain of salt.