Interpreting Data 101: Power Matters, and We Don’t Talk about It Enough
- Heather Duncan
- Jun 11, 2024
- 5 min read
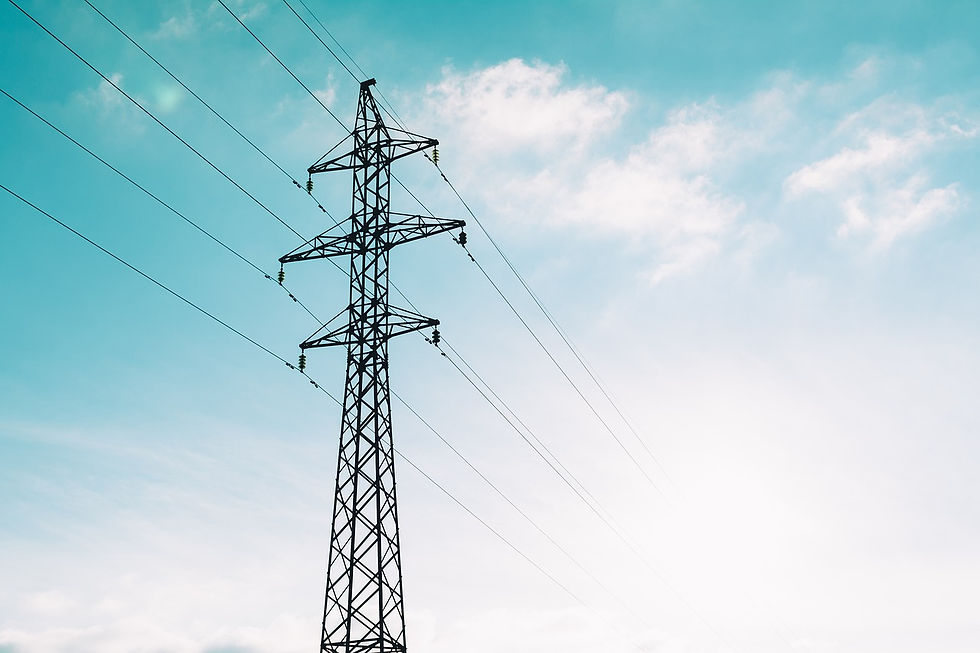
So far we have covered p-values and confidence intervals in our interpreting data series, two fundamental concepts essential to statistical data analysis. While p-values are often front and center in the results sections of published research studies, there’s an arguably more significant concept operating in the background: statistical power.
Recall that a p-value is a percent value reflecting the probability of observing the results generated by a study (or values more extreme than those observed) by chance, assuming our hypothesis is invalid. To illustrate, let’s say that we want to test the hypothesis that drug A will result in a decrease in blood pressure in patients being treated for hypertension, compared with patients with hypertension who are not being treated with drug A. This is known as the alternative hypothesis. It is also possible that drug A will not result in a reduction in blood pressure in hypertensive patients. This is known as the null hypothesis.
Now, on to statistical power. If we choose to reject the null hypothesis because our results achieve an acceptable level of significance based on a particular statistical test, power is the probability that we have correctly chosen to reject it, assuming the alternative hypothesis is in fact true.
This is a pretty powerful measure, pun intended. You might even be wondering why we focus so much on p-values and confidence intervals but rarely power. While conventions are not always logical, there are likely two reasons that power tends to fade into the background. The first is that while p-values are relatively straightforward to explain, power is a less intuitive concept. The second reason is that like significance, power is a value that must be set prior to even beginning a study (you can read about why post hoc power calculations are logically invalid in the article linked).
The calculation of power is directly related to effect size and sample size. Statistical power increases with sample size (number of participants) and effect size (the magnitude of the impact of the independent variable on the dependent variable), which is why sample size insufficiency is a common criticism levied at study design. Following the example above, if drug A has a relatively large effect size, then we would need a relatively smaller sample to achieve a desired power level (usually 80% by convention); but if drug A is only expected to reduce hypertension by a small amount, then we would need to observe its effect on a much larger sample to achieve our desired power.

To further explain, consider the difference between flipping a coin and rolling a twenty-sided die. We know that when flipping a coin, the probability of getting either heads or tails should be 50%, respectively. But if we roll a twenty-sided die, the probability of landing on any specific number should be 1 in 20, or 5%. If we wanted to prove that the coin really is fair and truly does display heads half the time, we could probably demonstrate that sufficiently in about ten or twenty flips. But if we wanted to prove that the odds of rolling a two on the twenty-sided die are truly 1 in 20, we would need many more trials than ten to sufficiently demonstrate our hypothesis simply because we would be less sure on any individual roll whether we were observing a true “effect” or random chance. Note that in this example, the audience’s expectations are a major factor in how large our sample needs to be (how many flips or rolls we choose to perform). While a die toss or a coin flip are low-stakes experiments, a drug trial is a much different situation. Therefore, effect and sample size, and by extension power, are often determined by how certain our audience wants to be that we have correctly rejected the null hypothesis.
When estimating effect size, researchers typically consider two things: the resources that are available to invest in the study, and the minimum effect size that would matter in context. The larger the effect size, the smaller the sample size required to achieve the desired power level and the fewer resources necessary to conduct the study. To illustrate, if drug A is shown to have a statistically significant effect on hypertension but only reduces it by about 2%, then one might question after the fact whether it was worth expending resources on a large sample to achieve the desired statistical power of 80% for such a small clinical payoff.
Sometimes it is relatively easy to determine the minimum effect size that would be “worth” detecting, and in these cases power and sample size determination are relatively straightforward calculations. But sometimes determining effect size can be trickier. For example, if the topic is new and there is relatively little extant literature, or if there is a high degree of uncertainty about what constitutes a meaningful effect, determining effect size can be challenging. This is especially true when baseline risk of experiencing a particular effect is not well understood.
For example, let’s say we want to test a drug that we believe may help prevent an infectious disease. If the baseline risk of being diagnosed with the disease in the absence of the intervention is not well established, as is often the case for infectious diseases, then it can be difficult to determine what effect size for a preventive intervention would “matter” in a clinical sense. If the participants are at high risk for acquiring a disease, then a relatively smaller effect size might be meaningful; but if the risk is relatively low, then we might not care so much about a relatively small effect size. The decision of where to set effect size and, by extension, sample size and power, often comes down to a judgment call on the part of the researcher. This is also why descriptive studies and pilot studies are essential to the work of public health science.
In summary, power is important to consider when evaluating a study because it informs us of some fundamental assumptions that the researchers chose to make in order to examine their effect of interest. A critique of the power of a study is a critique of these basic assumptions. And if these basic assumptions are invalid, then the p-values produced by the analysis do not actually tell us anything of use.
Although they are essential to study design, power calculations are typically not included in the main body of research articles. If power is a concern, it will usually only be alluded to in the limitations section, i.e. “our study may be underpowered to detect the effect of x on y.” Incidentally, studies can also be overpowered. An overpowered study wastes resources and can produce misleading results, particularly if the effect size is very small. Overpowering also artificially lowers p-values, which if done intentionally is considered a highly unethical practice. Intentional overpowering is a direct result of the undue emphasis placed on p-values in scientific research. Furthermore, different statistical tests require different power levels to be considered valid.
A more transparent approach to statistical power would mean that the public has a clearer picture of the way science works and the various constraints that factor into how research is performed. It would also shift emphasis away from p-values alone, the consequences of which has led to rampant p-hacking, a practice in which the researcher runs numerous statistical tests and manipulates the data in such a way that statistical significance is reached spuriously. For a fun illustration of how p-hacking works, check out this interactive dashboard from Nate Silver’s FiveThirtyEight blog.
The bottom line is that no study is perfect and statistics are as much an art as they are a science. They do not tell us objective truth and no one calculation should ever be relied upon as the sole measure of a study’s worth. But understanding power can help you to make better judgments about the strength and quality of a study’s results and less susceptible to being misled by unethical research practices.
Comments