Common Challenges for Data Analysts Using Sexual Orientation and Gender Identity (SOGI) Data
- Heather Duncan
- Feb 29, 2024
- 3 min read
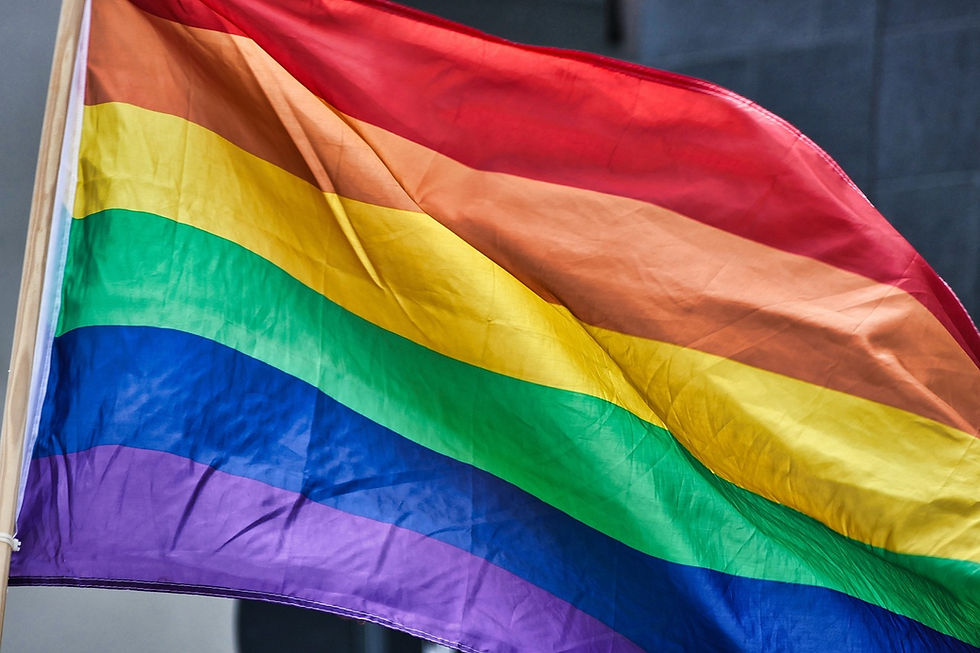
Sexual minorities are at greater risk for many health outcomes and are frequently the subject of public health research. Data on gender identity are almost always collected in studies involving human participants, and gender is frequently included as a variable in statistical analysis. Despite this, researchers frequently fail to consider the nuance involved in collecting and analyzing this type of data, leading to inaccurate or misleading results.
First, let’s quickly review the meaning of the terms “gender identity” and “sexual orientation.” Gender identity is a category distinct from biological sex, though the terms are often used interchangeably in scientific literature. Gender is a construct encompassing the social, behavioral, and cultural norms associated with being a “man” or “woman,” though it is not inherently binary. There is no biological basis for a person’s gender identity. Sexual orientation describes how an individual experiences sexual, emotional, and romantic attraction to others. Again, sexual orientation is often presented as a binary, but like gender, it exists on a spectrum.
Although the complexity of human gender and sexuality is now widely acknowledged, working with SOGI data can still present certain challenges to data analysts. Statistical analysis is a powerful tool, but it often requires the analyst to collect and code data in a way that reduces or even erases the distinctions between SOGI categories. Some common ways SOGI data present challenges to researchers include:
Intersectional identities–An individual may not fit neatly into the categories “gay” or “straight” just as they may not identify as “male” or “female.” Their identities may overlap or even defy existing categories. Consider, for example, the relatively recent recognition of non-binary individuals who do not identify as male or female, or the emergence of terms like “pansexual” that may overlap with existing categories like “bisexual.”
Open-ended response options–The “other” category with or without a write-in option is often used in surveys to capture additional information and allow for a broader range of responses. While a write-in category may be preferable because it allows for greater flexibility, coding these responses can be time-consuming and challenging. On the other hand, the “other” category without a write-in option only tells the analyst that the participant does not fit any of the categories supplied, and nothing more, leaving out potentially valuable information.
The need to split or lump responses–Coding variables for certain types of analysis often calls for categories to be disaggregated, or split, or for them to be aggregated, or lumped together. Splitting preserves complexity, but it can also create problems like small cell sizes which can bias the results of the analysis. Lumping erases complexity, but can potentially generate results that are easier to interpret or less prone to bias.
Changing language–Researchers must recognize that terms and meanings change over time and fall in and out of favor. When doing research on the experiences of sexual minorities, it is important to track which terms are preferred and why. Language that is out of date can discourage potential participants from agreeing to take part in your study and erode trust and credibility.
Culture–Not all cultures view gender, sex, and sexual orientation the same way. Consider, for example, the “two spirit” gender that exists in some Native American cultures but which is foreign to the western gender binary. In other cultures, sex and gender are not separable concepts, and this will color the way your participants respond to questions about gender identity. If your data will be collected in another language, it is vital that these concepts do not get lost in translation.
Despite these challenges, there are many strategies for overcoming them. If you are a public health researcher using SOGI data, you can read about best practices in the Urban Institute’s Do No Harm Guide. This free handbook covers many more challenges and methods for collecting, coding, and analyzing SOGI data effectively.
Comentários